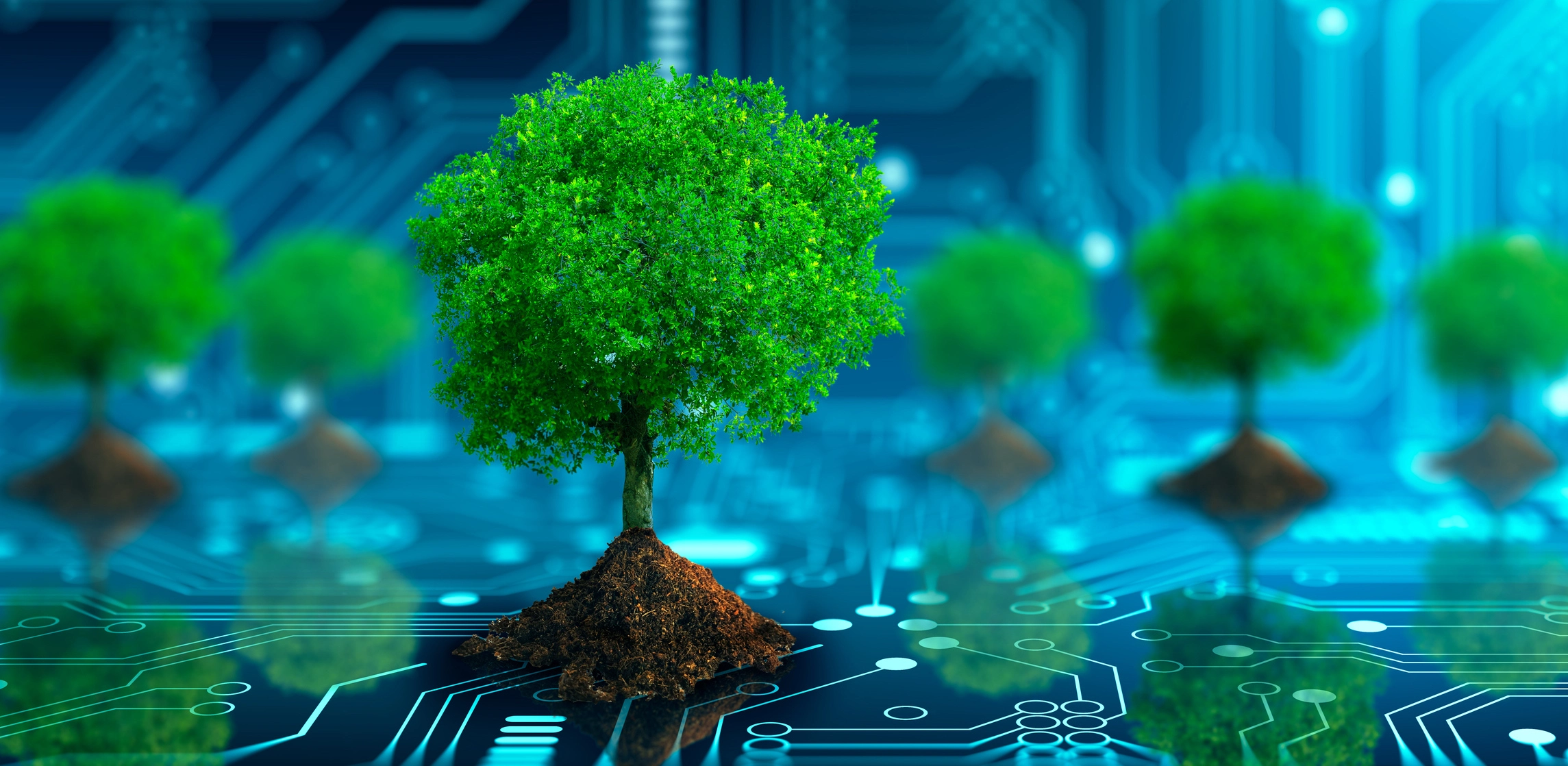
Generative AI has completely changed the way we interact with technology by enabling computers to generate human-like text, images, and more. While AI systems like ChatGPT and Google’s Bard lack a physical presence, their impact on the environment is far from negligible. These powerful AI systems are powered by networks of servers in data centers around the world, which require large amounts of energy and water to operate. Unfortunately, companies like OpenAI, Google, and Microsoft remain secretive about the water and energy usage of their AI models (Singh, 2023). As a result, the public remains in the dark about the extent of the environmental impact.
Due to their complexity, the training of AI models requires astronomical computational demands. A good example is OpenAI’s GPT-3, which was launched in 2020, and has been trained with a staggering 175 billion parameters (Korngiebel & Mooney, 2021). It is estimated that training such a large AI models can emit over 626,000 pounds of carbon dioxide equivalent emissions (Hao, 2019). This is equivalent to nearly five times the lifetime emissions of an average car in the United States (Hao, 2019). And this estimate does not even include the energy required to maintain these AI models! As AI’s influence continues to expand, the demand for more powerful machine learning models will only grow, leading to increased data usage and power consumption (Gartner, 2022). If we persist with our current AI practices, it is predicted that by 2030, the energy requirements for machine learning training, data storage, and processing could account for as much as 3.5% of global electricity consumption (Gartner, 2022).
While energy consumption is a significant concern, the water footprint of generative AI often remains overlooked. Data centers that power AI computations need substantial amounts of water for cooling and maintenance. Although exact figures remain unknown, a study suggests that training GPT-3 in Microsoft’s US data centres could have consumed 700,000 litres of fresh water (Li et al., 2023). Furthermore, to maintain the servers at optimal temperatures, a water bottle’s worth of fresh water is consumed for every 20 to 50 prompts given to the AI (Gendron, 2023).
Fortunately, the AI community is starting to embrace more sustainable practices in response to these environmental concerns. These sustainable practices include the use of specialized energy-efficient hardware, optimizing code or utilizing transfer learning techniques (Gartner, 2022).
In conclusion, generative AI tools have undeniably unlocked unprecedented capabilities. However, their ecological footprint is an issue that cannot be ignored. To reduce the environmental impact of generative AI tools, it is crucial to embrace green computing practices. In the ever-advancing world of AI, striking a balance between innovation and environmental stewardship is a necessity. We must ensure that as AI continues to thrive, it does so sustainably.
References:
Gartner. (2022, October 18). Gartner unveils top predictions for IT organizations and users in 2023. https://www.gartner.com/en/newsroom/press-releases/2022-10-18-gartner-unveils-top-predictions-for-it-organizations-and-users-in-2023-and-beyond
Gendron, W. (2023, April 14). ChatGPT needs to “drink” a water bottle’s worth of fresh water for every 20 to 50 questions you ask, researchers say. Business Insider. https://www.businessinsider.com/chatgpt-generative-ai-water-use-environmental-impact-study-2023-4?international=true&r=US&IR=T
Hao, K. (2020, December 7). Training a single AI model can emit as much carbon as five cars in their lifetimes. MIT Technology Review. https://www.technologyreview.com/2019/06/06/239031/training-a-single-ai-model-can-emit-as-much-carbon-as-five-cars-in-their-lifetimes/
Korngiebel, D. M., & Mooney, S. D. (2021). Considering the possibilities and pitfalls of Generative Pre-trained Transformer 3 (GPT-3) in healthcare delivery. Npj Digital Medicine, 4(1). https://doi.org/10.1038/s41746-021-00464-x
Li, P., Yang, J., Islam, M. A., & Ren, S. (2023). Making AI Less “Thirsty”: Uncovering and addressing the secret water footprint of AI models. arXiv (Cornell University). https://doi.org/10.48550/arxiv.2304.03271
Singh, M. (2023, June 14). As the AI industry booms, what toll will it take on the environment? The Guardian. https://www.theguardian.com/technology/2023/jun/08/artificial-intelligence-industry-boom-environment-toll