Almost in no time, AI-powered large language models (LLMs) such as ChatGPT, Bing AI Chat, Google Bard AI, etc., have gained popularity among the mainstream part of society. However, I have noticed increased social media attention, specifically among Latvian language speakers, about the lack of applicability and, oftentimes, even comedic outputs these language models create.
I test this observation by typing ‘write a poem’ in multiple languages in the dialogue interface of ChatGPT. English, Russian, French, Arabic, Hindi, Dutch, Latvian, Estonian and Lithuanian. Interestingly, although ChatGPT can produce, to some extent, coherent text in all prior languages, the latter three, i.e., the Baltic countries, excel with incoherent meanings and even grammar and style inconsistencies. Bang et al. (2023) argue that these are low-resource languages, i.e., languages with relatively few speakers. Not surprisingly, Latvian is spoken by 1,5 million native inhabitants (Latvian Presidency, 2023), and the AI model has not received the necessary data input to produce grammatically or style-wise coherent sentences (see picture).
So, how can this be an issue?
In Baltic countries, approximately 95% of individuals speak at least two languages (Latvian Presidency, 2015). The second language most often is Russian or English, i.e., high-resource languages.
This is a worry, as many native speakers might instead stick to high-resource languages while browsing or creating content. This, in return, reciprocates the poor usability of low-resource languages and exacerbates language polarization. The low-resource languages are, therefore, at risk unless new measures are implemented to better the LLM training, e.g., training AI with ‘small data’ as suggested by Ogueji, Zhu, Lin (2021), or feeding AI with new data resources.
Of course, the future of the language is not as one-dimensional and depends on many factors, but at times of language globalization, the mainstream AI tools have helped no further!
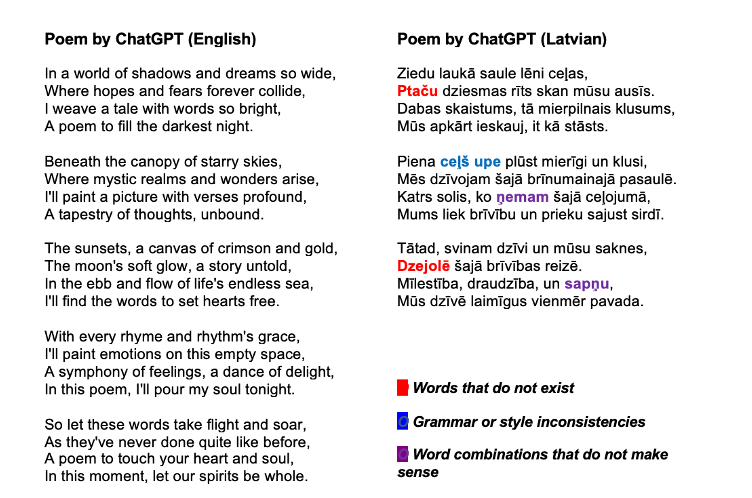
Bang, Y., Cahyawijaya, S., Lee, N., Dai, W., Su, D., Wilie, B., … & Fung, P. (2023). A multitask, multilingual, multimodal evaluation of chatgpt on reasoning, hallucination, and interactivity. arXiv preprint arXiv:2302.04023.
Ogueji, K., Zhu, Y., & Lin, J. (2021). Small data? no problem! exploring the viability of pretrained multilingual language models for low-resourced languages. In Proceedings of the 1st Workshop on Multilingual Representation Learning.
Latvian Presidency. (2015). Language. Latvian Presidency of the Council of the European Union. https://eu2015.lv/latvia-en/discover-latvia/language
What an important limitation of AI text generators that you are drawing attention to! I, as a German native speaker (which has a rather big amount of native speakers compared to Latvian, Estonian, and Lithuanian) have not encountered these difficulties on Chat GPT. It is truly important to protect language diversity and to encourage people to use AI text generators in their mother tongue rather than in English only. It seems also interesting that the English poem is almost twice as long as the Latvian. Is this a sign that the AI is somehow “aware” of the language barriers as it has less content to base its generation on? This might be an interesting idea for future research: comparing the output length across low and high-resource languages.
Very interesting blog! As a fellow speaker of a so-called “low-resource language”, I have also noticed that whenever using AI in Estonian, the output is often incredibly strange or just wrong, and it is much easier to just switch to a second language, such as English to get the results I wish. Unfortunately training the models is also very expensive, as I believe training GPT-3 alone was around 30 million, therefore to expand the usability of AI to smaller languages would require large investment. Perhaps in the future, institutions such as the EU could consider allocating money towards creating better and more diverse language models for AI technologies.
Very interesting blog! As a fellow speaker of a so-called “low-resource language”, I have also noticed that whenever using AI in Estonian, the output is often incredibly strange or just wrong, and it is much easier to just switch to a second language, such as English to get the results I wish. Unfortunately training the models is also very expensive, as I believe training GPT-3 alone was around 30 million dollars, therefore to expand the usability of AI to smaller languages would require large investment. Perhaps in the future, institutions such as the EU could consider allocating money towards creating better and more diverse language models for AI technologies.