Text to image AI is a groundbreaking form of Artificial Intelligence (AI) model that is able to generate a wide variety of images from natural language descriptions, or “prompts”, which are provided by users. Text to image generation is quickly becoming one of the most useful forms of AI, as demonstrated by the degree to which the technology’s ability to disrupt industries such as graphical design, content creation and the art/creative industry (Jones, 2022). Having used multiple prominent text to image generation models, I have noticed that the quality and usefulness of the overall outcome is often highly dependent on the quality of the prompt I provide – enter: Prompt Engineering.
Prompts are brief texts that instruct the AI on what the user wants to create. For text to image models, such as OpenAI’s DALL-E, details used to make these descriptions often include objects, shapes, colors, sizes, positions, actions and backgrounds. Prompt engineering, otherwise referred to as prompt design, is the practice of optimizing AI models for certain tasks by meticulously crafting the inputs given through adding certain phrases or specific instructions (Lawton, 2023).
In my experience, using text to image generation AI can be less intuitive to users starting out with the technology, as compared to pure language-based models such as ChatGPT. As a beginner, I noticed that I needed to use more specific language, over multiple iterations to even get close to the outcome I desired. Therefore, I have put together a list of tips aimed at helping beginners get the most out of these kinds of models through prompt engineering.
For the purpose of this blogpost, I will demonstrate the different outcomes from varying prompts using Bing Image Generator (powered by DALL-E 3) to generate an image of “a dog”. This is the result of the first prompt:
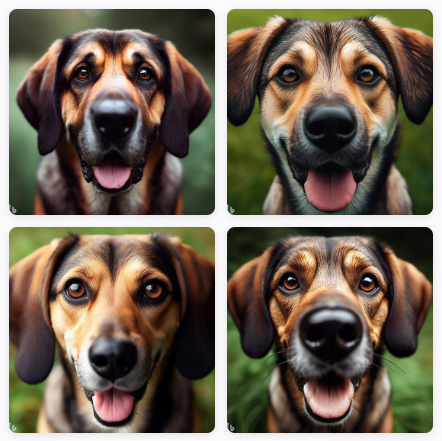
A first improvement would be to begin using keywords or phrases that are meaningful and relevant to the AI. For example, one could improve results by writing something like “a labrador”, yielding the following result:
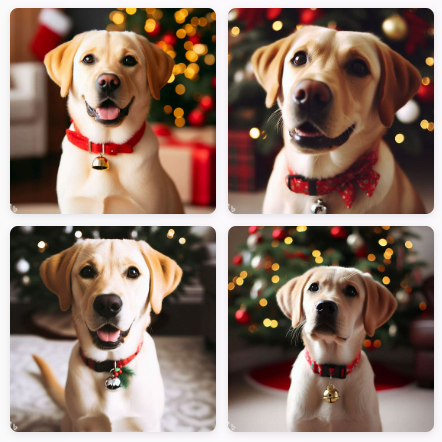
Similarly, one should use modifiers and qualifiers, which are adjectives, measurements, colors or numbers that modify/qualify the main subject of the prompt. Putting this into practice, one could prompt “a black labrador puppy” instead of “a labrador”, which generated the following images:
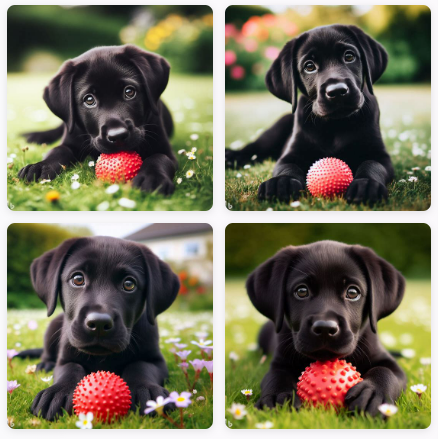
Moreover, the use of conditions and constraints can be an effective prompt engineering technique. This involves the use of locations, emotions, actions, orientations, or spatial relations that condition or constrain the main subject of the prompt. For example, instead of “a black labrador puppy”, one could describe “a black labrador puppy playing poker with other animals while wearing sunglasses”.
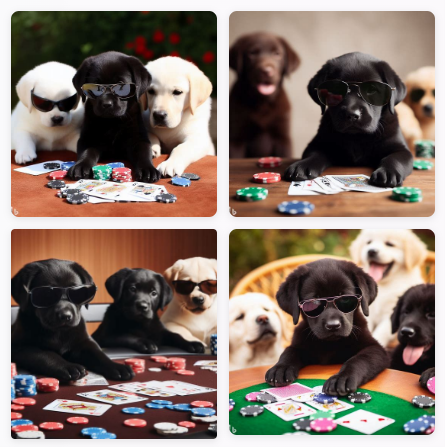
Lastly, one can provide style preferences to ensure that the images fit perfectly into the context in which they are being used. For example, if one wanted to use the images for a playful cartoon, one could use the prompt: “a black labrador puppy playing poker with other animals while wearing sunglasses, cartoon”. That input yielded these images:
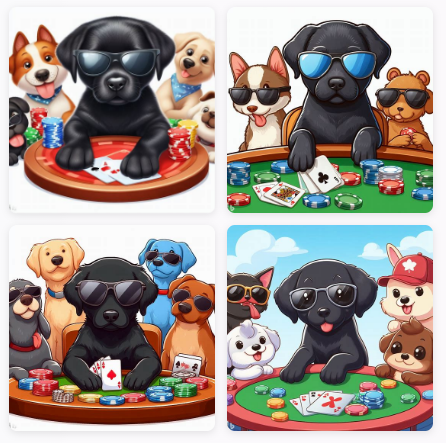
These examples show that, by simply improving the input given to an AI model, one can significantly increase the subjective usefulness and thus value of the AI model. Although it requires some time and experimentation to become proficient in prompt engineering, I believe everyone who uses any form of AI can gain massive utility from practicing this skill.
References:
Lawton, G. (2023). What is Prompt Engineering? – TechTarget Definition. Enterprise AI; TechTarget. https://www.techtarget.com/searchEnterpriseAI/definition/prompt-engineering
Jones, E. (2022, October 15). AI Image Generation: The End Of Creative Jobs? Geek Culture. https://medium.com/geekculture/ai-image-generation-the-end-of-creative-jobs-ca120ab2f64a