Last year, someone in my family began a marketing internship at a well-known FMCG company and shared a story about ChatGPT’s use there. At the time, ChatGPT and other generative AI tools were widely used. He told me that the company had stressed to its employees that they should never use company information in these AI tools because of the risk that sensitive data could be exposed or misused. Eventually, OpenAI sites were even blocked, frustrating many interns who used ChatGPT for translations on marketing materials.
Shortly after blocking these tools, the company developed its own proprietary AI model to ensure data privacy. While this approach was crucial for protecting confidential information, the model had limitations. It frequently mentioned the company’s name, exhibited bias towards the company, and some of the information it provided was simply inaccurate.
I looked into proprietary AI models and discovered that issues with bias in these systems have been ongoing for years, such as the bias found in Amazon’s AI recruiting tool back in 2018.¹ The tool, which was internally used by Amazon, was trained on resumes submitted to the company. However, since most of these resumes came from male applicants, the AI system developed a bias against women. This bias was not intentional, but it arose from the training data reflecting past gender imbalances in the tech industry. Amazon eventually had to stop using this AI tool due to its biased behavior.
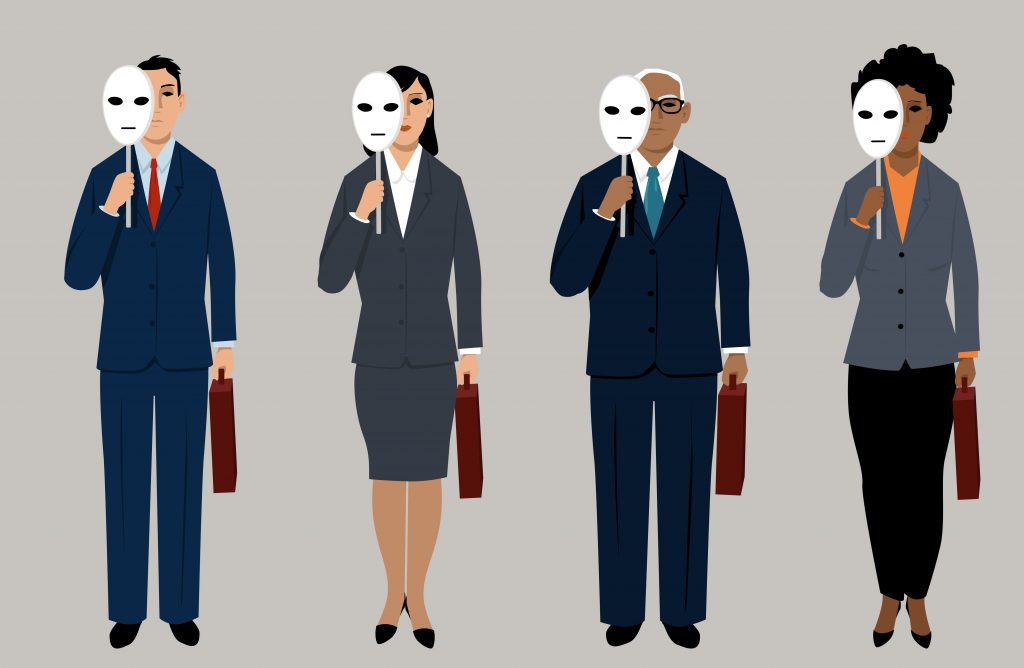
You can see that even with proprietary AI models designed to protect privacy, the biases it has underscore the benefits. A proprietary model is very different from an open-source AI, where the source code is available to the public. Because the source code is publicly available, people can benefit from a global community of developers who continuously improve the software. In contrast, proprietary AI models provide a higher level of security, with companies developing and controlling these models internally to ensure data privacy. This creates a discussion about the trade-offs between the benefits of community-driven innovation and the need for data protection. The question is, which approach ultimately better balances innovation and privacy?
Sources:
[0] Image from: https://www.datanami.com/2018/10/16/do-amazons-biased-algorithms-spell-the-end-of-ai-in-hiring/
This blog really raises an important question about whether the industry should prioritize the collaborative nature of open-source AI or the security and control of proprietary models. It seems like the best approach might be a hybrid one, balancing innovation with strict data governance. What are your thoughts on how companies might bridge the gap between these two approaches?
Thanks for your comment Frenk. I believe that a hybrid approach might work best, as long as the employees within a company are well informed about the risks of working with OpenAI tools and sharing sensitive data.