Demand forecasting
1Demand forecasting is nothing new within supply chain management. It involves forecasting future demand using statistical methods. Examples of traditional methods include: (weighted) moving averages, exponential smoothing, regression analysis, bottom-up and top-down forecasting (3SC, 2022). However, these methods are very time-consuming to maintain and rely on ‘manual’ processes. In addition, they are also limited with handling external variables such as weather, social media trends etc. An alternative will be discussed in this blog post.
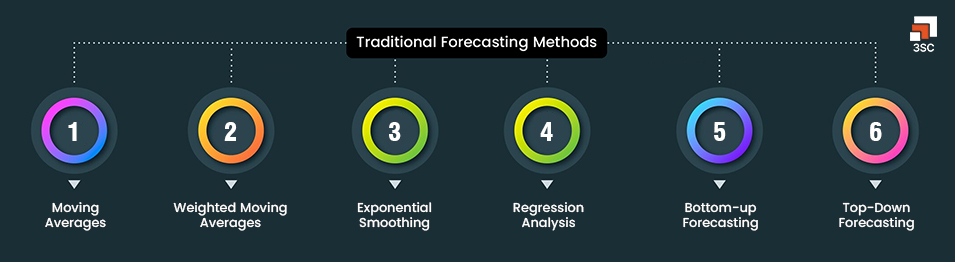
Machine learning as a solution
Machine learning can provide a solution to replace traditional forecasting methods. Machine learning models can adapt quickly to changes, providing real-time insights that drive smarter decision-making, minimize stockouts, and improve overall supply chain performance and efficiency. The advantage of a machine learning model is that it learns by doing: The longer the model is used, the more accurate the predictions will be. But this also means that the model needs massive amounts of data to train itself. The image below shows some data source examples for a machine learning model. This amount of variables is complex to include in manual forecasting methods.
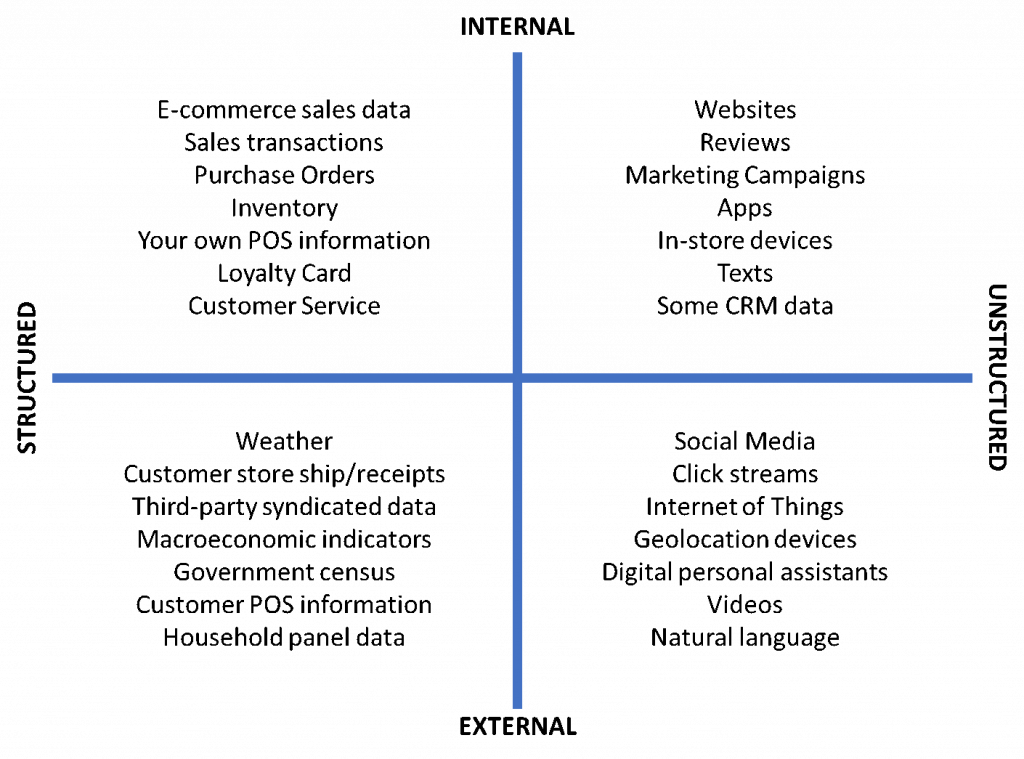
Is machine learning able to outperform traditional demand forecasting methods
Machine learning (ML) techniques have been explored in supply chain management since the early 2000s. At that time, however, ML methods showed no significant improvement over traditional forecasting methods (Carbonneau et al., 2007). Today, the situation is different. Recent studies demonstrate that ML methods now outperform traditional techniques (Feizabadi, 2020). Additionally, a comprehensive literature review has confirmed that ML frequently delivers better results than conventional methods (Aamer et al., 2021). This is mainly due to larger amounts of (usable) data and improved algorithms.
My view for the future
I personally think machine learning forecasting is the future within supply chains. Even though traditional methods are reasonably accurate. Machine learning methods have the ability to include immense amounts of data and variables that traditional methods could never include. In my opinion, the biggest obstacle to large-scale machine learning application lies in the transparency of the entire supply chain. Today’s supply chains are so complex that insight into an entire supply chain is very difficult. But this transparency is needed to feed machine learning models. After all, a model is only as good as the data it consumes. When this supply chain transparency is enhanced, e.g. by large-scale block chain application, the world lies open for large-scale machine learning application, allowing global supply chains to operate more efficiently (and thus often more sustainably).
Literature
3SC. (2022, September 12). Overview Of Demand Forecasting Techniques and Impact Of AI/ML On It. https://3scsolution.com/insight/demand-forecasting-techniques-and-planning
Aamer, A., Yani, L. P. E., & Priyatna, I. M. A. (2021). Data Analytics in the Supply Chain Management: Review of Machine learning applications in demand Forecasting. Operations and Supply Chain Management an International Journal, 1–13. https://doi.org/10.31387/oscm0440281
Carbonneau, R., Vahidov, R., & Laframboise, K. (2007). Machine Learning-Based demand Forecasting in supply chains. International Journal of Intelligent Information Technologies, 3(4), 40–57. https://doi.org/10.4018/jiit.2007100103
Feizabadi, J. (2020). Machine learning demand forecasting and supply chain performance. International Journal of Logistics Research and Applications, 25(2), 119–142. https://doi.org/10.1080/13675567.2020.1803246
Wilson, E. W. (2019, August 26). Forecaster’s & Planner’s Guide To Data. IBF. https://demand-planning.com/2019/08/26/forecasting-data-types/
I really enjoyed this post and the way it highlights the evolution of demand forecasting through machine learning. I agree that while traditional methods have been reliable, the complexity of today’s supply chains demands more dynamic solutions like ML, which can adapt in real-time to external factors like weather and social trends.
Your point about transparency being key for ML models to reach their full potential is thought-provoking. As supply chains become more transparent—possibly with technologies like blockchain—the ability to integrate massive data sets into these models will transform efficiency and sustainability in global operations. Great insights!