In the dynamic world of technology, it feels like a new Generative AI tool is launched nearly every day. Whether it’s generating images, writing text, or assisting with marketing strategies, the possibilities are vast. However, with so many tools available, it can be overwhelming to keep up with what works, what doesn’t, and how to extract the most value from each.
GenAI holds enormous potential, but the quality of its output varies significantly depending on both the AI tool and its user. Often, the issue isn’t the AI itself, but rather how users frame their queries or provide input. As I learned during my studies at EUR, the principle of “trash in, trash out” (TITO) applies heavily. For example, while ChatGPT can provide high-quality solutions for tasks like “identifying empty packages on a conveyor belt,” the output is highly dependent on asking the right, specific questions—like “how to identify them as cheaply and quickly as possible.”
One of the limitations of GenAI is that it lacks the lateral thinking humans naturally employ. This can make it difficult for AI to propose creative or unconventional solutions without direct hints. For instance, a recruiter on LinkedIn shared a story where a salary negotiation was resolved by switching the applicant from a full-time to a part-time role while accepting the proposed salary, a compromise that AI might not suggest unless explicitly asked (See screenshots below).
Despite its power, GenAI often falls short of meeting academic standards. When writing scholarly texts or seeking qualitative references, the output is frequently inadequate unless very specific sources are provided upfront. This can be frustrating when using technologies that promise high efficiency but don’t deliver the expected depth in research or precision in content creation.
Moreover, crafting high-quality content—whether it’s text or images—requires more than simply using AI tools. Many users, including myself, would benefit from formal training on how to maximize the capabilities of these technologies. For example, I used GenAI to create a digital product, handling everything from translations and marketing text to generating SEO keywords and ad images through tools like ChatGPT and Imagen via Canva. While it was incredibly efficient for marketing, other aspects of the process were more challenging, and my success depended at some points on my prior business experience and knowledge, ChatGPT wouldn´ have. Another positive experience with GenAi: During my Marketing Research course, I had to learn the R Studio program. Whenever I or even the professor encountered challenges while solving tasks, I turned to ChatGPT for assistance, and it proved to be a great help. The AI provided basic solutions and saved me a lot of effort by guiding me through problems with RStudio efficiently.
In my experience, the learning curve for effectively using GenAI can be steep, and I often found it easier to complete some tasks manually rather than spending time configuring the tools. Additionally, there’s the issue of trust. When GenAI generates unfamiliar information, I frequently feel the need to double-check the accuracy and reliability of the sources, which diminishes the time-saving benefits AI is supposed to offer.
In conclusion, while GenAI is a groundbreaking tool with the potential to revolutionize industries, it is not yet a perfect substitute for expertise, particularly in academic or specialized contexts. Users, myself included, need better training and guidance to achieve optimal results, and trust in the technology remains an ongoing challenge. Maybe a business idea hint given here?
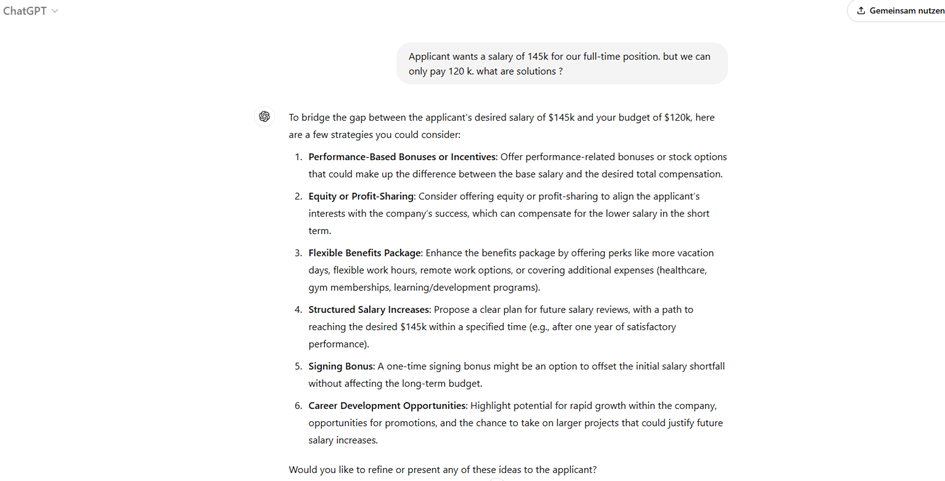
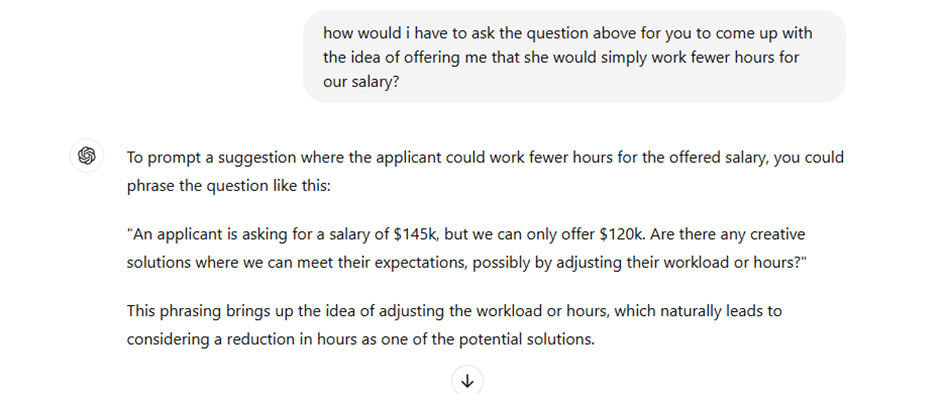