I recently came across Artificial Intelligence Virtual Artist (AIVA) which helps create music and offers a lot of customizations. As someone who has always wanted to make music, but has never been able to learn any instruments, this offered an avenue to a lot of possibilities, and I wanted to see what AI can offer us. Hence, I made an account on AIVA and was quite fascinated by the various options it provided.
Firstly, it allowed composing a track in the following ways:
- You could provide it with a style which could vary from lo-fi, techno, sync-wave, etc., duration and the number of compositions and it will create new music for you.
- You could provide it with a chord progression or upload an already existing one. It also suggests a chord progression, or you can give it a prompt to generate one. (The prompt was very fascinating as I provided it with an overview, and it gave a decent chord progression.)
- A step-by-step process where you could customize everything from styles, chord progressions, composition layers, instruments, provide prompts.
- Upload an influence or an existing music file

I started with providing it with a simple style to create a small lo-fi playlist as this is the kind of music I listen to a lot while working, and it was able to give a quick 30 second clip which was probably not the best lo-fi track but still better than I expected.
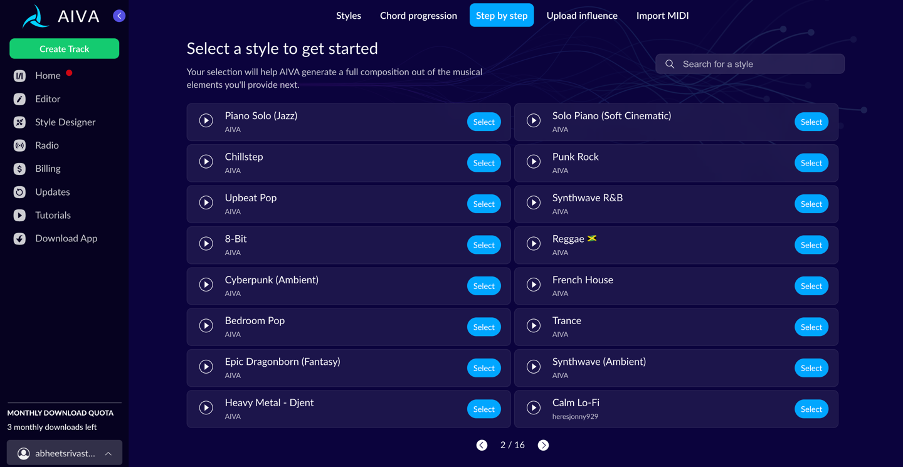
Next, I tried giving it more details and seeing what the AI could when provided with more details. I used the chord progression function, and it allowed me to generate a chord progression using a prompt. I provided it with the prompt to use the same chord progression as used in the song, “Sound of Silence” by Simon and Garfunkel. The results were quite fascinating. While the chord progression wasn’t exactly copied, but still the music generated took me by surprise and was worth listening to.
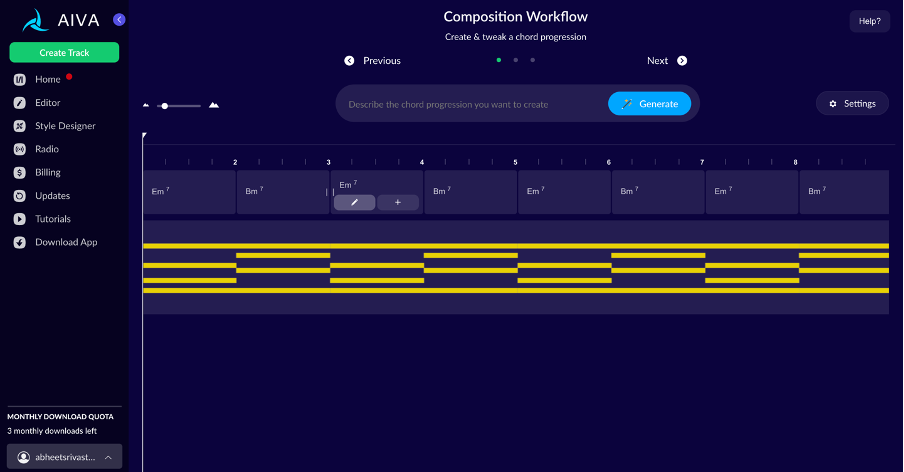
Lastly, I experimented with other genres and more detailed experiments using Daft Punk as the style, and asking the AI to generate a fast-tempo Daft Punk styled song, and I was actually able to get quite an interesting song that was worth listening to. It allowed working with composition layers, changing song durations, and also generating multiple tracks.
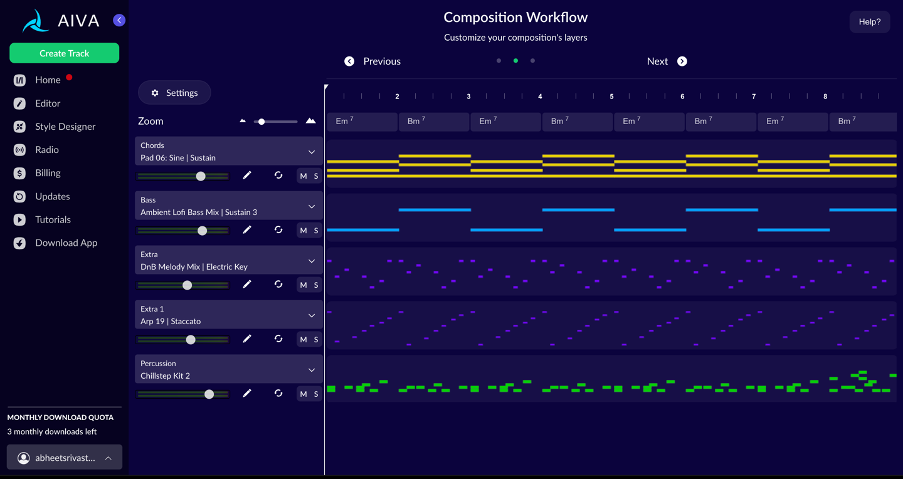
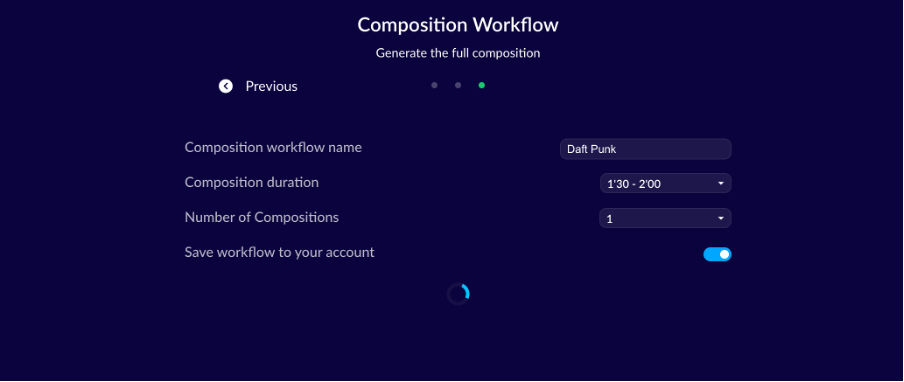
Experimenting with AIVA was quite fun and if not for the limit on their free subscription, I would have experimented more. I believe that with adding more customised and user-friendly interfaces where someone with minimal music knowledge could generate music clips through mere prompts, customization around instruments and also adding your own music and mixing and matching, GenAI could really transform the creative process of music making. It would also be interesting if we could compare human produced music and AI generated music side by side and see the similarities and differences.
We are living in exciting times, and it would be interesting to see how the future evolves, and how the human and machine made music combines to evolve the creative process.