Many blog articles and conversations around Artificial Intelligence (AI) and more specifically Large Language Models evolve around ChatGPT and for a good reason. However, through an architecture like Retrieval Augmented Generation short RAG, the performance of Large Language Models (LLMs) can be vastly improved, making it especially interesting for company applications. RAG functions as an information retrieval system acting between the LLM like ChatGPT and the enterprise content like documents, images or audio. Based on the input prompt, only the relevant snippets of context out of the whole database are fetched and provided to ChatGPT to ground the response. This leads to increased accuracy and can furthermore be used as a stand-alone solution without input from outside data. (HeidiSteen, 2023)
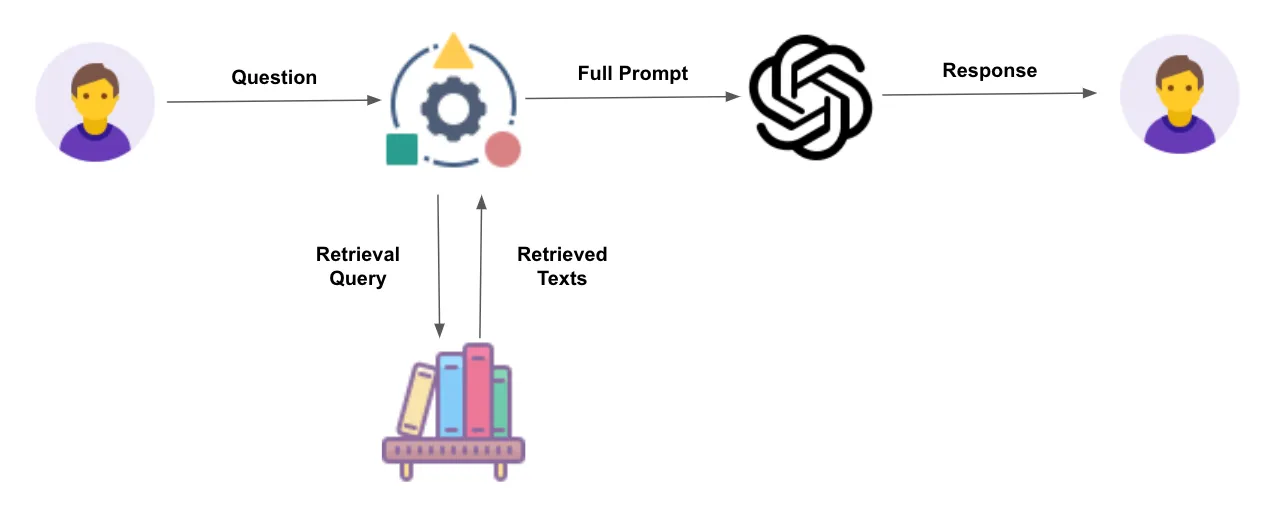
RAG can therefore transform companies access to their own data, by leveraging this knowledge to generate more relevant responses for employees or even customers. Additionally, this reduces common drawbacks of LLMs like hallucinations or lack of domain-specific knowledge (Jiang et al., 2023). The great part here is that through Microsoft’s partnership with OpenAI, all OpenAI models, like gpt-35-turbo or gpt-4 are embedded into the Azure ecosystem. The Azure OpenAI ecosystem allows users to ground ChatGPT models with RAG, through the Azure Cognitive Search service with just a few clicks, given that the data is stored in one of Azures cloud solution like Data Lake v2, Blob storage or an SQL database. (Balapv, 2023)
From my own experience of implementing such a solution to answer thousands of support tickets every week with the use of a gpt-35-turbo model grounded in a extensive FAQ database, the results are quite impressive, outperforming well trained human support agents. Even role-based access is possible, allowing for a differentiation in access levels to different types of company documents.
In conclusion, LLMs like ChatGPT are just the beginning and augmented with the right architectures like RAG in the right infrastructure solution like Microsoft Azure, companies can quickly transform their access to their own data and even build business applications. Through the service Microsoft 365 Copilot which will be available to the general public this November, the RAG method using GPT-4 will come standard for every account. Giving each user these tools throughout the whole Microsoft suit including apps like OneDrive, Outlook and Teams. I would appreciate your experience with Microsoft’s AI solutions and possibly you own thoughts or even projects with Retrieval Augmented Generation.
References
Balapv. (2023, July 31). Retrieval Augmented Generation using Azure Machine Learning prompt flow (preview) – Azure Machine Learning. Microsoft Learn. https://learn.microsoft.com/en-us/azure/machine-learning/concept-retrieval-augmented-generation?view=azureml-api-2
Barak, N. (2023, July 19). Information Retrieval For Retrieval Augmented Generation | Towards AI. Medium. https://pub.towardsai.net/information-retrieval-for-retrieval-augmented-generation-eaa713e45735
HeidiSteen. (2023, September 13). RAG and generative AI – Azure Cognitive Search. Microsoft Learn. https://learn.microsoft.com/en-us/azure/search/retrieval-augmented-generation-overview
Jiang, Z., Xu, F. F., Gao, L., Sun, Z., Liu, Q., Dwivedi-Yu, J., Yang, Y., Callan, J., & Neubig, G. (2023). Active Retrieval Augmented generation. arXiv (Cornell University). https://doi.org/10.48550/arxiv.2305.06983