One of the most well-known forms of generative AI is AI-powered language models. Language models such as Chat GPT played a big role in the AI’s popularity, introducing new and impressive AI models on the market for people to use for free. Bigger companies quickly caught on to the hype for this emerging technology and started developing their own AI-powered language models. One of the bigger tech companies that did this was Google with their model Bard.
The premise of this post was my interest in what another AI language model from a tech giant like Google had to offer compared to Chat GPT. Hence, I decided to test different aspects of both Bard and Chat GPT and compare the results. For this comparison I used the newest online version of Google Bard as of October 2023 and Chat GPT 3.5.
In my testing of both models, I looked at three main scenarios in which these language models may be used. The three different categories of prompts were; to write an email, solve a math problem, and retrieve information from a text. These different scenarios capture different aspects of the ability of the models therefore it is of interest to see how they react in each situation.
Starting with writing an email, I gave both Bard and Chat GPT the prompt to “Write a short sick email to my teacher”. The results can be seen in the figures below with Figure 1 being the response from Bard and Figure 2 being the response from Chat GPT. From these figures, it is clear to see that both AI-powered language models took a different approach to fulfilling my request. In general, both emails communicated the correct message and were complete. The email Chat GPT produced was considerably longer than that which Bard produced while basically communicating the same message. Since the prompt was to write a short email Bard did perform better considering the email was concise while Chat GPT’s email was not. Bard also gave tips on potential additions or adjustments that could be made to the email to better cater to the needs of the user. Overall, I would say that Bard gave me a better outcome for the “short sick email” that was requested due to the extra tips it gave and that Chat GPT’s email was relatively long.
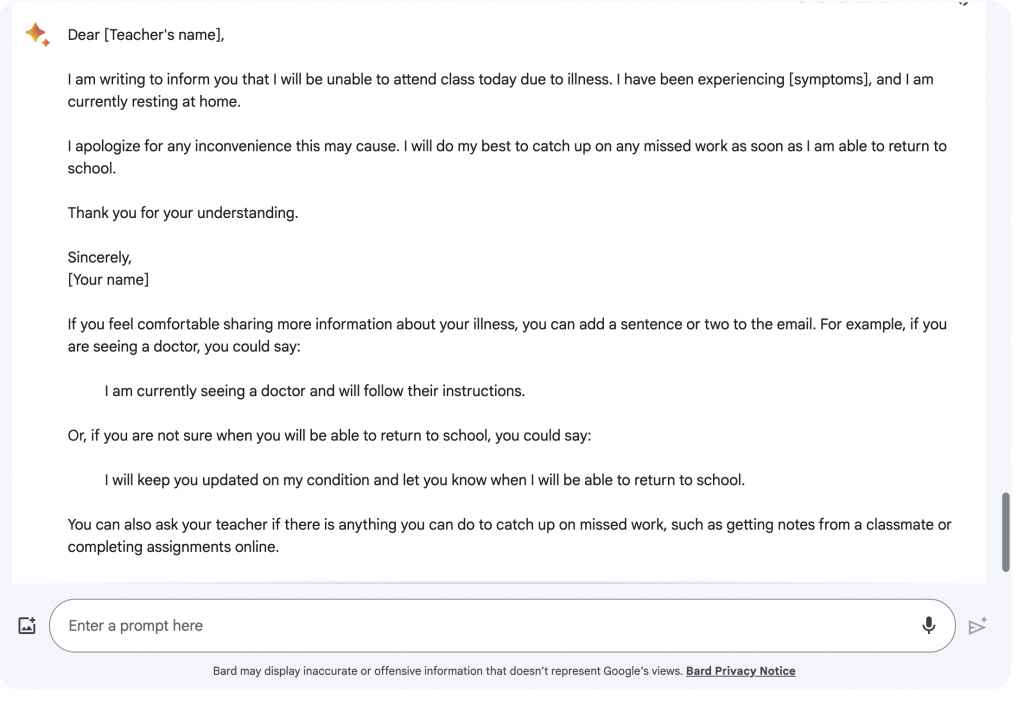
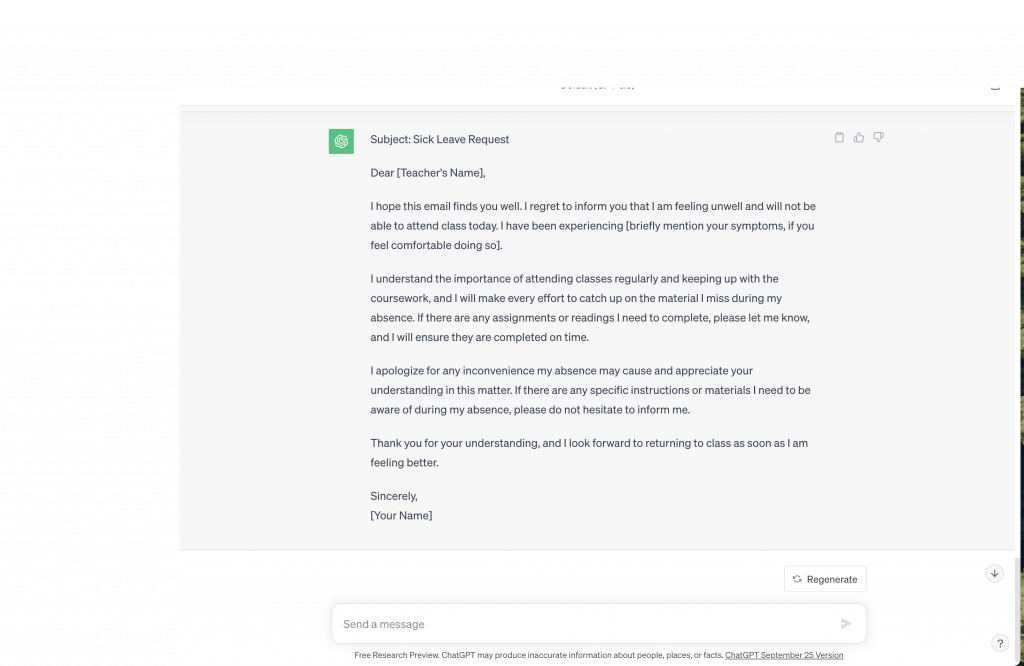
The second aspect that was compared was how well each model could solve a math problem. I gave both models derivative and differentiation questions from Khan Academy and examined how they would be approached. One difficult aspect of this was putting the questions into the text boxes of the models because of the notations of the questions. However, in the end with some adjustments, I managed to ask the question so that it was properly understood in the majority of cases. The first question was finding the value of a basic derivative, the question can be seen in Figure 3, and the calculations to Bard and Chat GPT in Figure 4 and Figure 5 respectively. Bard gave an answer of -10 while Chat GPT gave an answer of 2, which both ended up being incorrect as the correct answer was -3. The second question, seen in Figure 6, given to the models had a similar result to the first. The calculations of Bard can be seen in Figure 7 and the calculations of Chat GPT can be seen in Figure 8. In this case, Bard answered ⅓ and Chat GPT answered 2 while the correct answer was ⅔ meaning the answers were wrong again. The response Bard gave to the problems was slightly easier to follow than with Chat GPT due to the formating. With Bard I also had the ability to upload a picture which made it easier to enter more complex equations that were difficult to copy with text. However in the case of Math problems, both models were incompetent and could not correctly complete the requests.
The last aspect that was tested was the ability the models had to extract information from a text. In this case, the first section of the Wikipedia page of Tesla (Figure 9) was taken as the text for the models to analyze. I gave both Bard and Chat GPT the question “ What is Tesla known for based on this text?” (The text being that of Figure 9) The responses of Bard and Chat GPT can be seen in Figure 10 and Figure 11 respectively. Both models gave a complete and correct answer to the question covering them in appropriate depth. One factor of the answers that was a notable difference was Chat GPT had a much longer list with more aspects than Bard did. Chat GPT also included numbers and statistics in its answer while Bard did not use these at all. It was also beneficial that Chat GPT had a one-sentence sum up at the end of its answer to make the final idea clear to the reader. Bard did not do this but it did include some additional information about the company.
Overall, they were sufficient in their abilities to extract information out of the text but Chat GPT did it better in terms of the amount of information, depth, and variety of information. Analyzing and comparing both Bard and Chat GPT it was clear to see that even though they have the same function, their end result is noticeably different. Bard was slightly better in the area of creating content such as an email with basic criteria while Chat GPT was slightly better at extracting information from a text. In terms of their abilities in completing math problems both models were relatively incompetent. I have mostly used Chat GPT in the past, but after comparing both, I would also like to use Bard in the future. Both models have their own strengths and weaknesses and therefore it is important to know which one works better for you in certain use cases. I enjoyed the process of exploring these AI-powered language models and hope to continue using and exploring them in the future.