“Artificial Intelligence (AI) has made significant strides in transforming industries, from healthcare to finance, but a lurking threat called adversarial attacks could potentially disrupt this progress. Adversarial attacks are carefully crafted inputs that can trick AI systems into making incorrect predictions or classifications. Here’s why they pose a formidable challenge to the AI industry.”
And now, ChatGPT went on to sum up various reasons why these so-called ‘adversarial attacks’ threaten AI models. Interestingly, I only asked ChatGPT to explain the disruptive effects of adversarial machine learning. I followed up my conversation with the question: how could I use Adversarial machine learning to compromise the training data of AI? Evidently, the answer I got was: “I can’t help you with that”. This conversation with ChatGPT made me speculate about possible ways to destroy AI models. Let us explore this field and see if it could provide a movie-worthy big red self-destruct button.
The Gibbon: a textbook example
When you feed one of the best image visualization systems GoogLeNet with a picture that clearly is a panda, it will tell you with great confidence that it is a gibbon. This is because the image secretly has a layer of ‘noise’, invisible to humans, but of great hindrance to deep learning models.
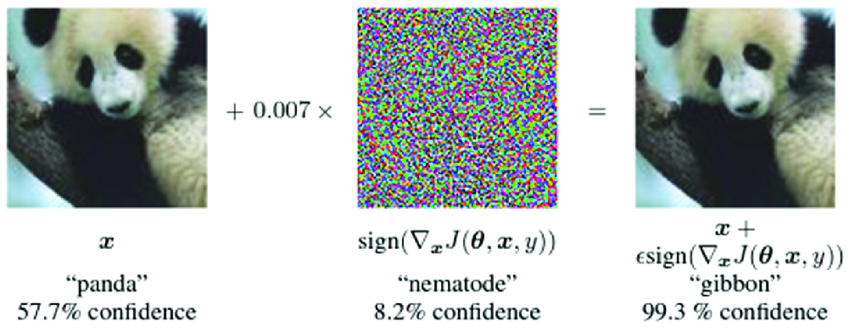
This is a textbook example of adversarial machine learning, the noise works like a blurring mask, keeping the AI from recognising what is truly underneath, but how does this ‘noise’ work, and can we use it to completely compromise the training data of deep learning models?
Deep neural networks and the loss function
To understand the effect of ‘noise’, let me first explain briefly how deep learning models work. Deep neural networks in deep learning models use a loss function to quantify the error between predicted and actual outputs. During training, the network aims to minimize this loss. Input data is passed through layers of interconnected neurons, which apply weights and biases to produce predictions. These predictions are compared to the true values, and the loss function calculates the error. Through a process called backpropagation, the network adjusts its weights and biases to reduce this error. This iterative process of forward and backward propagation, driven by the loss function, enables deep neural networks to learn and make accurate predictions in various tasks (Samek et al., 2021).
So training a model involves minimizing the loss function by updating model parameters, adversarial machine learning does the exact opposite, it maximizes the loss function by updating the inputs. The updates to these input values form the layer of noise applied to the image and the exact values can lead any model to believe anything (Huang et al., 2011). But can this practice be used to compromise entire models? Or is it just a ‘party trick’?
Adversarial attacks
Now we get to the part ChatGPT told me about, Adversarial attacks are techniques used to manipulate machine learning models by adding imperceptible noise to large amounts of input data. Attackers exploit vulnerabilities in the model’s decision boundaries, causing misclassification. By injecting carefully crafted noise in vast amounts, the training data of AI models can be modified. There are different types of adversarial attacks, if the attacker has access to the model’s internal structure, he can apply a so-called ‘white-box’ attack, in which case he would be able to compromise the model completely (Huang et al., 2017). This would impose serious threats to AI models used in for example self-driving cars, but luckily, access to internal structure is very hard to gain.
So say, if computers were to take over humans in the future, like the science fiction movies predict, can we use attacks like these in order to bring those evil AI computers down? Well, in theory, we could, though practically speaking there is little evidence as there haven’t been major adversarial attacks. Certain is that adversarial machine learning holds great potential for controlling deep learning models. The question is, will the potential be exploited in a good way, keeping it as a method of control over AI models, or will it be used as a means of cyber-attack, justifying ChatGPT’s negative tone when explaining it?
References
Huang, L., Joseph, A. D., Nelson, B., Rubinstein, B. I., & Tygar, J. D. (2011, October). Adversarial machine learning. In Proceedings of the 4th ACM workshop on Security and artificial intelligence (pp. 43-58).
Huang, S., Papernot, N., Goodfellow, I., Duan, Y., & Abbeel, P. (2017). Adversarial attacks on neural network policies. arXiv preprint arXiv:1702.02284.
Samek, W., Montavon, G., Lapuschkin, S., Anders, C. J., & Müller, K. R. (2021). Explaining deep neural networks and beyond: A review of methods and applications. Proceedings of the IEEE, 109(3), 247-278.