Have you ever taken hours browsing around supermarkets searching for the most nutritious food options? Did it take you too much time figuring out which recipes to cook best, with the groceries you bought? Struggles when dealing with food are numerous, starting from choosing products with appropriate nutrients simply not knowing enough recipes.
Therefore, we introduce NutriNet – a mobile application that is going to make shopping and meal planning easier!
• Grocery shopping & meal planning simplification:
NutriNet simplifies grocery shopping and meal planning, by analyzing which products and recipes fit the users’ desired grocery item wishes, nutrition values and store preferences best. NutriNet aims to address the challenges being implicit to personalized nutrition and helps consumers make healthier food choices by simplifying the grocery shopping and meal planning process. This shall be done by eliminating the need to perfectly understand all nutrition values or to search for numerous recipes. NutriNet completes all these tasks for you in real-time and provides clear and accessible recommendations for you.
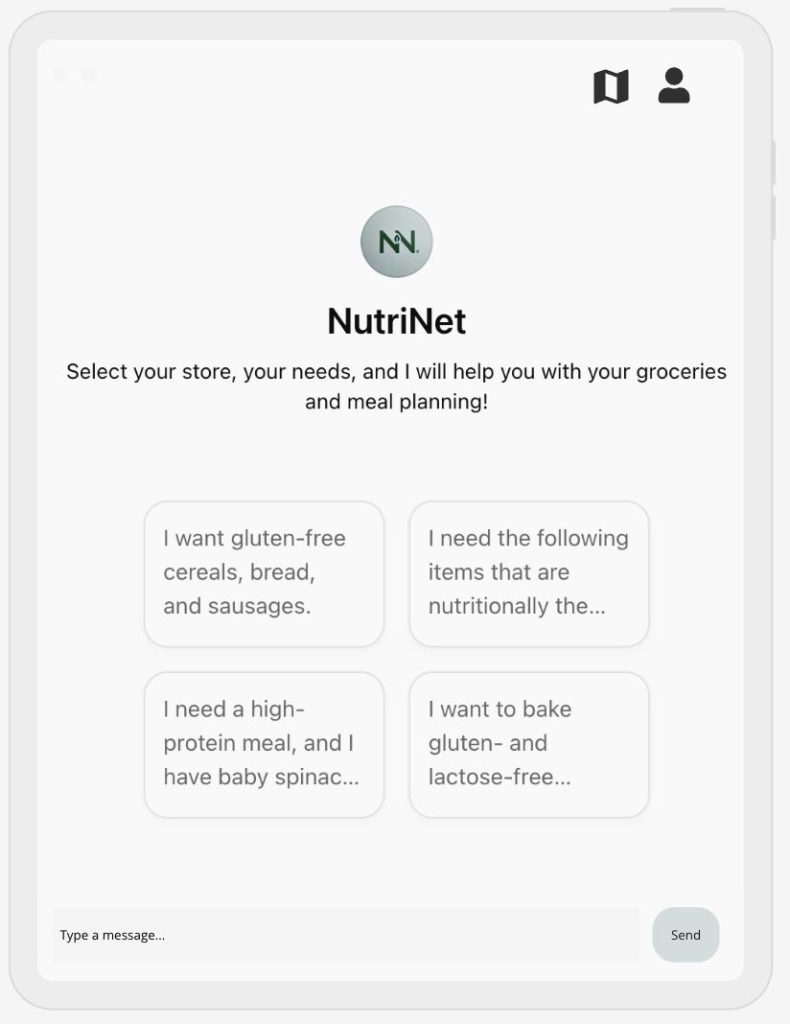
• Real-time personal assitant: NutriNet acts as a multifunctional application providing value to consumers by solving various food related problems in real-time. Appearing as a chatbot, it is aimed at taking general grocery shopping lists or meal wishes as input query, combined with preferences for food characteristics (e.g., nutrients, allergies) and grocery stores. It then provides brand specific and personalized grocery shopping lists, as well as meal recommendations, if so desired. Moreover, grocery items can also be added into the initial grocery shopping list query, by scanning them with the integrated AR tool. The product will then be detected visually and thus will be integrated into the shopping list input query.
• Long-term customer engagement: NutriNet distinguishes from competition by providing personalized and customized advice. This is possible, as NutriNet consists of a database, having incorporated stock and product information of the major supermarkets in the Netherlands. In contrast, classic applications, which try to meet similar needs (e.g., meal recommendation) rather focus on counting nutrients for the purpose of short-term weight loss, instead of personalizing grocery shopping lists and meal recommendation to enable a healthier lifestyle for users. Those applications are usable on short term but are proven to have low adherence over the time (Chen et al., 2015).
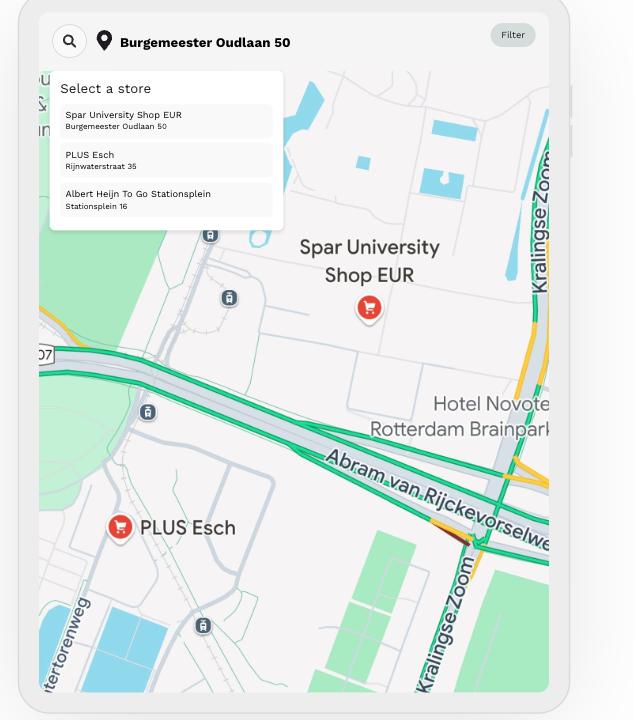
• Personalized recommendations: NutriNet leverages generative AI to offer accurately personalized recommendations. Users can simply enter their preferences, while prompting a grocery list or a meal, such as gluten-free or high in protein, and the generative AI powered application will provide accurately personalized results.
However, personalization and raising awareness for healthy foods are not the only purposes of NutriNet. It also addresses sustainability issues that supermarkets are facing. By gathering consumer purchase and search data in the application, consulting services can be offered to supermarkets, enabling them to plan ordering and stockholding processes more efficient. Hence, supermarkets should be able to reduce food waste due to overstocking on long-term.
NutriNet offers a 2-sided network platform, yielding important value to both, consumers as well as supermarkets.
Contributors
574051 – Duong Dao
728070 – David Wurzer
738898 – David Do
562387 – Roxi Ni
References
Chen, J., Berkman, W., Bardouh, M., Ng, C. Y. K., & Allman-Farinelli, M. (2019). The use of a food logging app in the naturalistic setting fails to provide accurate measurements of nutrients and poses usability challenges. Nutrition, 57, 208-216.