As this course draws to a close, I want to use this opportunity to continue my series on AI-based hiring tools and inform you of one other AI-based hiring tool that you may encounter in the near future: HireVue. If you haven’t already, please refer to my first blogpost about Pymetrics for more context on this series.
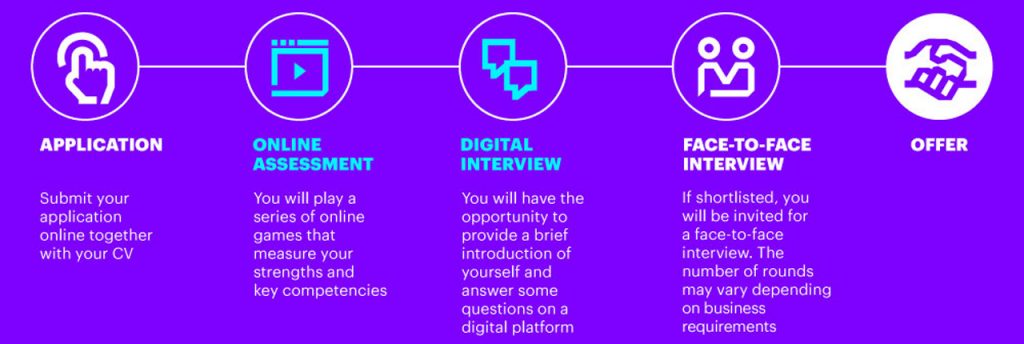
Assuming you have passed the online assessment stage (Pymetrics) in Figure 1, you will now advance to the digital (on-demand) interview stage. Most companies that follow this same structure, will be using the HireVue platform to conduct this interview.
What is HireVue?
HireVue is a software company that provides video interviews for the company you applied to. In this blog we will be focusing on their on-demand interview. In their on-demand interview, candidates are asked pre-determined questions (by the company) using the HireVue platform. Candidates record their answers to these questions, which are consequently submitted to the company.
Here is where it gets interesting: your recorded answers are not evaluated by a human recruiter, but by AI. HireVue uses voice and facial recognition software in order to analyze your answers and assign you a score. This score is then used to rank you amongst other applicants. More concretely, you are ranked based on your facial expressions, eye contact and movements, body language, tone, and keywords in your recorded answers.
How does the on-demand interview look like?
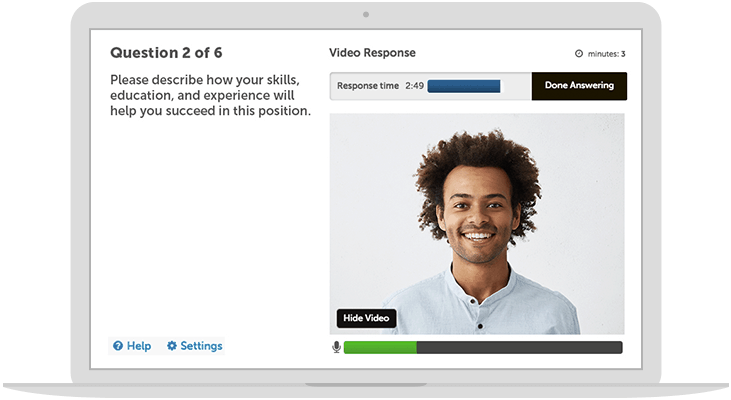
Figure 2 depicts a candidate that is in the midst of recording an answer to one of the pre-determined questions. Prior to a question appearing, a candidate will have 30 seconds to think about what he/she wants to say. The company you are applying to can decide whether they allow for retries (in case you mess up your answer). If they do, the number of attempts is usually communicated in the beginning of the interview (during my own personal experience with HireVue I had three attempts to answer a question).
Hirevue vs. Pymetrics
HireVue was introduced in the recruitment process for reasons similar to Pymetrics: to leverage (faster) AI-driven predictions that allow for increased diversity and mitigated bias when selecting applicants.
However, in contrast to Pymetrics, HireVue’s algorithm has faced large waves of public criticism. AI researchers have frequently voiced their concerns, claiming HireVue’s technology is ‘profoundly disturbing’. The criticism reached its boiling point late 2019, when prominent rights group Electronic Privacy Information Center (EPIC) filed an official complaint with the Federal Trade Commission, urging them to investigate HireVue and its business practices. In response, HireVue announced in January 2021 that it would stop relying on facial recognition to assess their job candidates. However, they will continue to analyze other biometric data, including speech, intonation and behaviour.
Voice your opinion!
Having read the above, where do you stand in regard to HireVue? Would you welcome such an interview? Especially considering HireVue interviews may be used at the very company you have your sights set on (Accenture, JP Morgan, Goldman Sachs, Morgan Stanley, etc.).
References
Did this blog pique your interest? Please refer to the used sources below for more in-depth coverage on this topic:
- https://www.accenture.com/th-en/careers/local/recruiting-in-the-new
- https://www.hirevue.com/blog/hiring/video-interviewing-guide
- https://www.topinterview.com/interview-advice/what-is-a-hirevue-interview
- https://searchhrsoftware.techtarget.com/definition/HireVue
- https://cultivatedculture.com/video-interviews
- https://www.washingtonpost.com/technology/2019/10/22/ai-hiring-face-scanning-algorithm-increasingly-decides-whether-you-deserve-job
- https://epic.org/2021/01/hirevue-facing-ftc-complaint-f.html