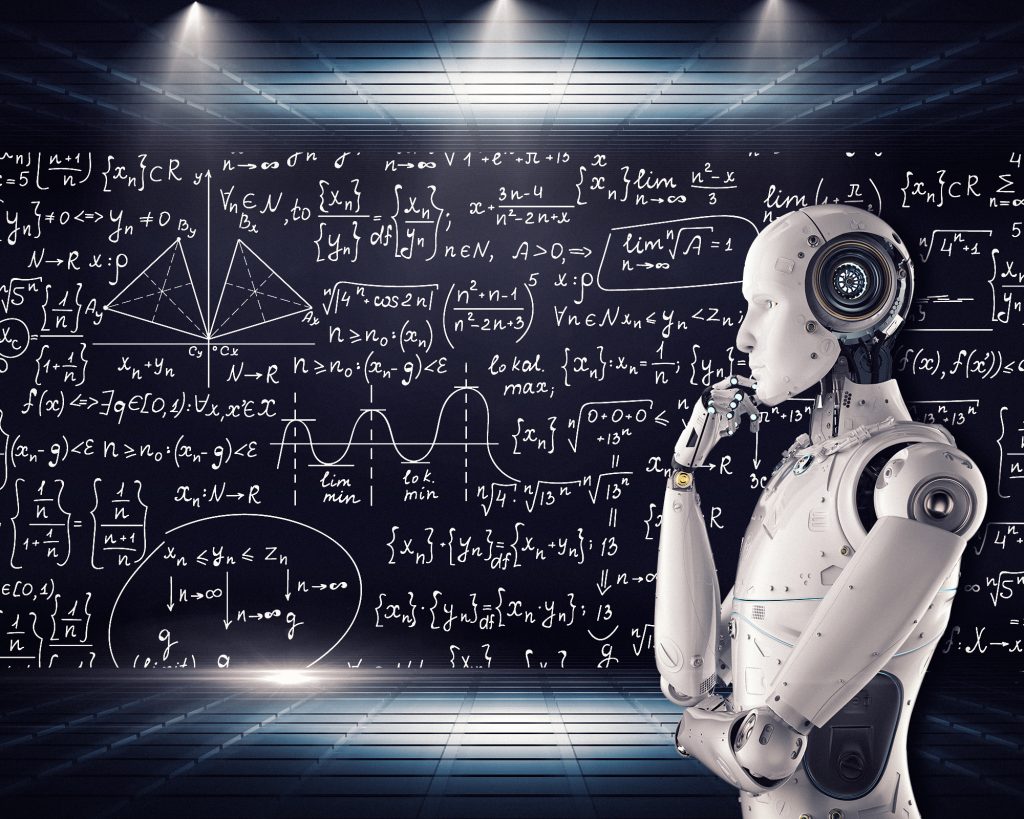
The number of processes that are being taken over by Artificial Intelligence (AI) is rapidly increasing. Additionally, the results of these processes are not solely to assist humans in their decision-making process anymore: the results yielded by the algorithm contain the decision, more often than not. In these cases, if there is a human being involved in the process, he/she most of the time simply needs to adhere to the results of the algorithm (Bader & Kaiser, 2019).
This brings up the accountability question: in case the algorithm misperforms, who is accountable for the consequences? The most logical options are either the designers/ creators of the algorithm or the users of the algorithm. However, both options don’t seem to have an immediate preference over the other, since both possibilities raise a lot of potential difficulties.
Firstly, assessing accountability at the designers or creators of the algorithm raises concerns. One of the first scientists to be concerned about accountability in the use of computerized systems was Helen Nissenbaum. In 1996, much ahead of her time, she wrote a paper in which she described four barriers that obscure accountability in a computerized society. These four barriers are rather self-explanatory: many hands, bugs, computer as scapegoat, and ownership without liability (Nissenbaum, 1996). To this day, these four barriers very well illustrate the difficulty to designate accountability when a process is aided by (or even fulfilled by) an algorithm (Cooper et al., 2022).
Secondly, placing responsibility on the user is difficult, as, in a significant proportion of the cases, the user has zero to very little influence on the content of the algorithm. Also, as stated before, users are sometimes obliged to adhere to the outcome presented to them by the algorithm (Bader & Kaiser, 2019).
Currently, most case studies show that the creators of the algorithms sign off their accountability to the users during the acquisition of the product containing the algorithm. For example, when buying a Tesla with ‘Full Self-Driving Capability’, Tesla simply states that these capabilities are solely included to assist the driver and that therefore, the driver is responsible at all times (Tesla, 2022; Ferrara, 2016).
In my opinion, it would be wise to explore the gap in the possibilities of what can be done to not only legally (as Tesla does), but also morally, sign-off accountability to the users of the algorithm. Maybe already during the design phase of the algorithm. A proposed research question that could be addressed can be stated as follows:
“What can be done about the design of an artificially intelligent algorithmic system to maintain accountability on the user side?”
References
- Bader, V., & Kaiser, S. (2019). Algorithmic decision-making? The user interface and its role for human involvement in decisions supported by artificial intelligence. Organization, 26(5), 655–672. https://doi.org/10.1177/1350508419855714
- Cooper, A. F., Laufer, B., Moss, E., & Nissenbaum, H. (2022). Accountability in an Algorithmic Society: Relationality, Responsibility, and Robustness in Machine Learning. ArXiv:2202.05338 [Cs]. http://arxiv.org/abs/2202.05338
- Ferrara, D. (2016). Self-Driving Cars: Whose Fault Is It? Georgetown Law Technology Review, 1, 182.
- Nissenbaum, H. (1996). Accountability in a computerized society. Science and Engineering Ethics, 2(1), 25–42. https://doi.org/10.1007/BF02639315
- Tesla. (2022). Autopilot and Full Self-Driving Capability. https://www.tesla.com/support/autopilot