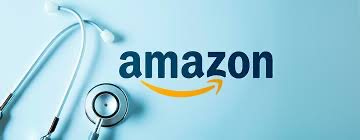
Amazon, the household name for e-commerce companies, has recently considered to enter the industry of healthcare. Amazon’s resources, which includes data platforms spread all over the world have identified individuals’ patterns in shopping and web searching. This feature consisting of personal health may be able to recognize a health risk for the patient and help them to get the fastest medical help they need. From getting online appointments with a doctor through Alexa to ordering medicine based on your health data. Getting this scenario may not be only a dream but a reality.
Not only could Amazon make an impact in healthcare, but it is also conceivable for them to disrupt it. Amazon could for instance change how prescriptions are made. For example, with the supply chain, the supply chain of Amazon could have a lot of options to deliver medicine or prescriptions to their customers, through delivery or even pick-ups at stores. This instead of patients having to come to the pharmacy itself to get their medicine. Not only that, but with voice assistants like Alexa. Doctors could use audio to fill in prescription orders instead of writing an administrating it on paper or computer. These data integration abilities streamline everything for patients, especially for members that have amazon prime. This way Amazon Prime could offer lower prices for medicine, because their membership with prime, can lower medical costs for consumers because of the Prime subscription (El Khatib, 2022). While Amazon, may be a small player at first in healthcare. Their distribution strategies could be crucial into becoming a dominant contender in the industry of healthcare.
Furthermore, Amazon has a lot of potential due to having the ability to offer product recommendations that could be personalized. Recently, Amazon had integrated Whole foods into their ecosystem, which could enhance consumer data. Healthcare data combined with personalized data, could make Amazon capable of offering the right recommendations for patients for things like, medicine, food or advice that could treat patients better or living a better life. In addition, with the technological advancement of the algorithm’s amazon has, they will be capable of detecting certain patterns in purchases and the health of patients that are very sick or terminally ill. These algorithms could provide reports and care to help patients getting better health and curing illness (Provost & Murray, 2022).
Amazon possesses an array of many technologies. for instance, Alexa and Prime are the main fundamental aspects into shifting Amazon from the e-commerce industry to combining e-commerce and healthcare. Fast delivery services and assistants like Alexa, might make it feasible for consumers from rural or developing areas to obtain the right healthcare. Patients for instance that live in places far away from the city may be able to use amazon to arrange appointments or order medicine. Furthermore, echo, another important feature of Amazon, has the possibility to streamline communication between consumers and hospitals. Another example of features amazon has is patients being able to use notifications to take medicine, this could strengthen the care for patients and lower costs of medical costs (Cutler, 2007), because there will not be a break in consistency due to possible forgetfulness. All these innovations could not only help the patients better but also create a strong loyalty of the brand for Amazon. When all said and done, it raises the question. Is Amazon going to disrupt the healthcare industry, is it going to survive against the competitors and will Amazon be able to provide healthcare for those in need?
Cutler, D. M. (2007). The lifetime costs and benefits of medical technology. Journal of health economics, 26(6), 1081-1100.
El Khatib, M., Hamidi, S., Al Ameeri, I., Al Zaabi, H., & Al Marqab, R. (2022). Digital disruption and big data in healthcare-opportunities and challenges. ClinicoEconomics and Outcomes Research, 563-574.
Provost, L. P., & Murray, S. K. (2022). The health care data guide: learning from data for improvement. John Wiley & Sons.