Together with Machine Learning, Artificial Intelligence (or: AI) can be considered one of if not the hottest emerging innovations in the field of technology nowadays (Duggal, 2021). AI entails the ability of a computer or a machine to ‘think by itself’, as it strives to mimic human intelligence instead of simply executing actions it was programmed to carry out. By using algorithms and historical data, AI utilizes Machine Learning in order to comprehend patterns and how to respond to certain actions, thus creating ‘a mind of its own’ (Andersen, n.d.).
History
Even though the initial days of Artificial Intelligence research date back to the late 1950s, the technology has just recently been introduced to the general mass on a wider scale. The science behind the technology is complex, however AI is becoming more widely known and used on a day-to-day basis. This is due to the fact that computers have become much faster and data (for the AI to derive from) has become more accessible (Kaplan & Haenlein, 2020). This allows for AI to be more effective, to the point where it has already been implemented in every-day devices i.e. our smartphones. Do you use speech or facial recognition for unlocking your phone? Do you use Siri, Alexa or Google Assistant? Ever felt like advertisements on social media resonate a bit too much with your actual interests? Whether you believe it or not, it is highly likely that both you and I come into contact with AI on a daily basis.
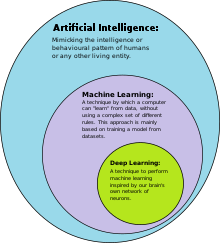
That’s good… right?
Although the possibilities for positively exploiting AI seem endless, one of the more recent events which shocked the world about the dangers of AI is a phenomenon called ‘deepfaking’. This is where AI utilizes a Deep Learning algorithm to replace a person from a photo/video with someone else, creating seemingly (!) authentic and real visuals of that person. As one can imagine, this results in situations where people seem to be doing things through media, which in reality they have not. Although people fear the usage of this deepfake technology against celebrities or high-status individuals, this can – and actually does – happen to regular people, possibly you and I.
Cybercrime
Just last month, scammers from all over the world are reported to have been creatively using this cybercrime ‘technique’ in order to commit fraud against, scam or blackmail ordinary people (Pashaeva, 2021). From posing as a wealthy bank owner to extract money from investors, to blackmailing people with videos of them seemingly engaging in a sexual act… as mentioned before, the possibilities for exploiting AI seem endless. Deepfakes are just another perfect illustration of this fact. I simply hope that, in time, the positives of AI outweigh the negatives. I would love to hear your perspective on this matter.
Discussion: Deepfake singularity
For example, would you believe this was actually Morgan Freeman if you did not know about Artificial Intelligence and deepfakes? What do you think this technology could cause in the long term, when the AI develops itself into a much more believable state? Will we be able to always spot the fakes? What do you think this could lead to in terms of possible scamming or blackmailing, if e.g. Morgan Freeman were to say other things…?
References
Duggal, N. (2021). Top 9 New Technology Trends for 2021. Available at: https://www.simplilearn.com/top-technology-trends-and-jobs-article
Andersen, I. (n.d.). What Is AI and How Does It Work? Available at: https://www.revlocal.com/resources/library/blog/what-is-ai-and-how-does-it-work
Kaplan, A., & Haenlein, M. (2020). Rulers of the world, unite! The challenges and opportunities of artificial intelligence. Business Horizons, 63(1). https://doi.org/10.1016/j.bushor.2019.09.003
Pashaeva, Y. (2021). Scammers Are Using Deepfake Videos Now. Available at: https://slate.com/technology/2021/09/deepfake-video-scams.html