In today’s business landscape, data is key. The widespread adoption of Artificial Intelligence (AI) and Machine Learning (ML) has sparked a compelling debate about the future of the data scientist’s role. As we progress into an era where being code literate and possessing technical knowledge are no longer barriers to entry, the accessibility of generative AI tools has transcended the boundaries of academic research and tech giants. It’s now within reach for anyone with an internet connection and an email address.
It made me wonder if this transformation means the death of data scientist-like professions or whether it signifies the birth of new roles. I will delve into this question using my personal experience as a starting point.
The Learning Curve
About a year ago I started in a newly created role as a junior business controller. I quickly realized the importance of harnessing data to create insightful dashboards that guide data-driven decision-making. Learning to navigate the complex world of data was a challenge in itself (I mean, how do I know that I can trust my data? What data is available and what is relevant?), but the real game-changer came when I discovered the power of generative AI.
It was like having a 24/7 coding mentor at my fingertips
Starting from scratch with SQL was no walk in the park. I encountered countless errors, syntax hiccups, and moments of sheer frustration. But I followed some courses, watched YouTube videos and found out that SQL is amazing (honestly: I would highly recommend learning it)! It was during this process that I stumbled upon an invaluable ally: ChatGPT. Whenever I hit a roadblock, I would turn to ChatGPT and put my code-related questions in. Within seconds, I’d receive clear and concise explanations, troubleshooting tips, and even code snippets to resolve my issues. It was like having a 24/7 coding mentor at my fingertips.
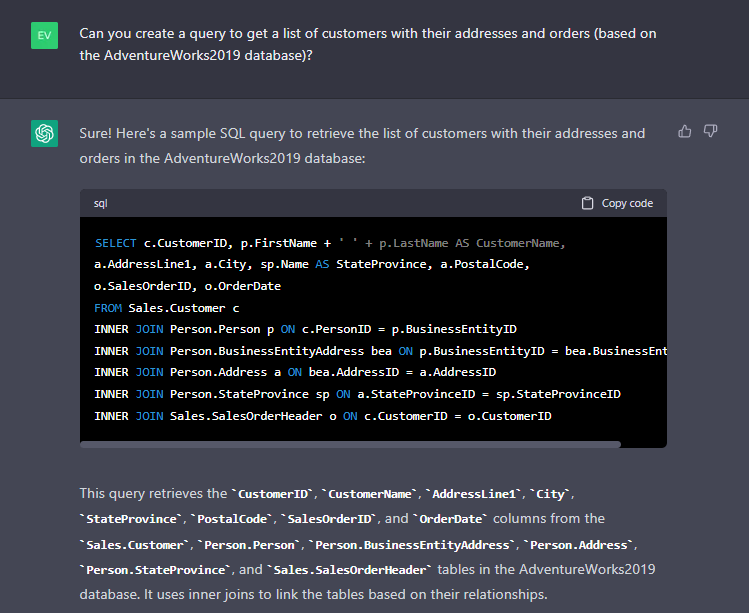
The Role of Generative AI in Data-Related Work
Generative AI, like ChatGPT, is not just a tool for beginners like me. I’m convinced that it has the potential to revolutionize the way data scientists and coders work. But should we see it as a friendly companion or a sneaky enemy that is stealing jobs?
AI as a Productivity Booster
First and foremost, generative AI can significantly enhance the productivity of data professionals. It excels at automating repetitive tasks, such as data retrieval and cleaning as well as tackling error messages. This frees up valuable time for more critical analysis. It can also serve as a valuable learning resource, providing instant answers and explanations for coding queries. Due to this I have experienced a steep learning curve in my SQL journey.
Human data scientists and coders bring a unique skill set to the table; they possess the ability to interpret, contextualize, and make nuanced decisions based on data
The Future of Data-Related Jobs
The future of data-related jobs stands at a crossroads, where the demand for AI-ready professionals who can effectively harness tools like ChatGPT is already evident in job descriptions. The fear of AI replacing human expertise is a legitimate concern, but it’s important to remember that AI is a tool, not a replacement. While generative AI offers substantial productivity gains, it’s not immune to errors or hallucinations, reminding us of the irreplaceable value of deep domain expertise. Human data scientists and coders bring a unique skill set to the table; they possess the ability to interpret, contextualize, and make nuanced decisions based on data. AI can undoubtedly assist in the technical aspects of the job, streamlining processes and automating tasks, but it cannot replicate the human touch required for holistic and insightful data analysis.
Well, the way I see it..
In my role, generative AI has truly been a game-changer. It’s become an ally helping me out with coding challenges and making my dashboards way better and more trustworthy. It surprised me by getting results faster than I expected, and I can’t complain about that! And whenever my SQL query was actually running properly, I put it in and asked for suggestions to improve performance. Using ChatGPT for my coding-related questions has boosted my efficiency and effectiveness, and I see it as a sneak peek into the future of data work. It’s all about humans and AI teaming up, playing to each other’s strengths for some seriously awesome outcomes.
So, the next time you encounter a coding conundrum, don’t hesitate to turn to generative AI. It’s not a replacement for your expertise but a trusted partner in your data-driven journey.
Curious to hear your thoughts and experiences! Also let me know whether some of you use other GAI tools for your data- and coding-related issues! 😉