We all know ChatGPT since the whole technological frenzy that happened in 2022. This computer program was developed by OpenAI using GPT-3.5 (Generative Pre-trained Transformer) architecture. This program was trained using huge dataset and allows to create human-like text based on the prompts it receives (OpenAI, n.d.). Many have emphasized the power and the disruptive potential such emerging technology has whether it be in human enhancement by supporting market research and insights or legal document drafting and analysis for example which increases the efficiency of humans (OpenAI, n.d.).
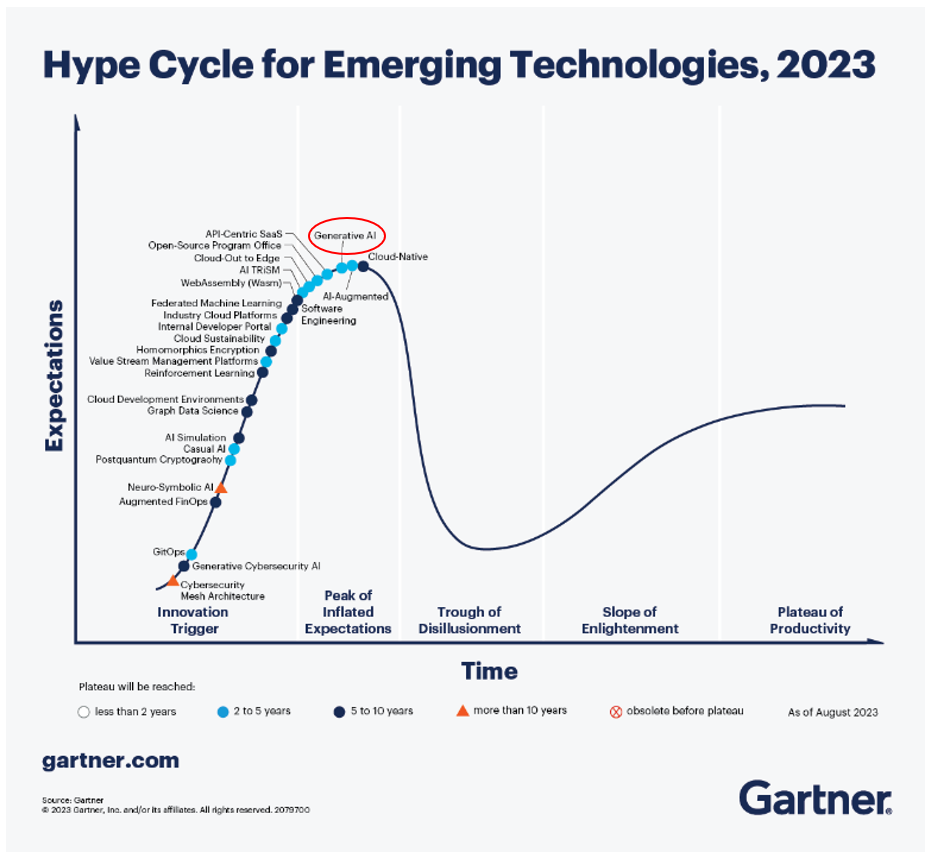
Hype cycle for Emerging Technologies retrieved from Gartner.
However, despite its widespread adoption and the potential generative AI has, there are still many limits to it that prevent us from using it to its full potential. Examples are hallucinating facts or a high dependence on prompt quality (Alkaissi & McFarlane, 2023; Smulders, 2023). The latter issue links to the main topic of this blog post.
I have asked in the past to ChatGPT, “can you create diagrams for me?” and this was ChatGPT’s response:
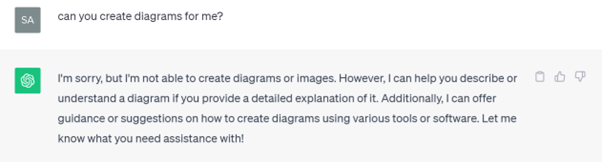
I have been using ChatGPT for all sorts of problems since its widespread adoption in 2022 and have had many different chats but always tried to have similar topics in the same chat, thinking “Maybe it needs to remember, maybe it needs to understand the whole topic for my questions to have a proper answer”. One day, I needed help with a project for work in understanding how to create a certain type of diagram since I was really lost. ChatGPT helped me understand but I still wanted concrete answers, I wanted to see the diagram with my own two eyes to make sure I knew what I needed to do. After many exchanges, I would try again and ask ChatGPT to show me, but nothing.
One day came the answer, I provided ChatGPT with all the information I had and asked again; “can you create a diagram with this information”. That is when, to my surprise, ChatGPT started creating an SQL interface, representing, one by one, each part of the diagram, with the link between them and in the end an explanation of what it did, a part of the diagram can be shown below (for work confidentiality issues, the diagram is anonymized).
It was a success for me, I made ChatGPT do the impossible, something ChatGPT said itself it could not provide for me. That day, ChatGPT outstripped its limitations for me. This is how I realized the importance of prompt quality.
This blog post shows the importance of educating the broader public and managers about technological literacy in the age of Industry 4.0 and how with the right knowledge and skills, generative AI can be used to its full potential to enhance human skills.
Have you ever managed to make ChatGPT do something it said it couldn’t with the right prompt? Comment down below.
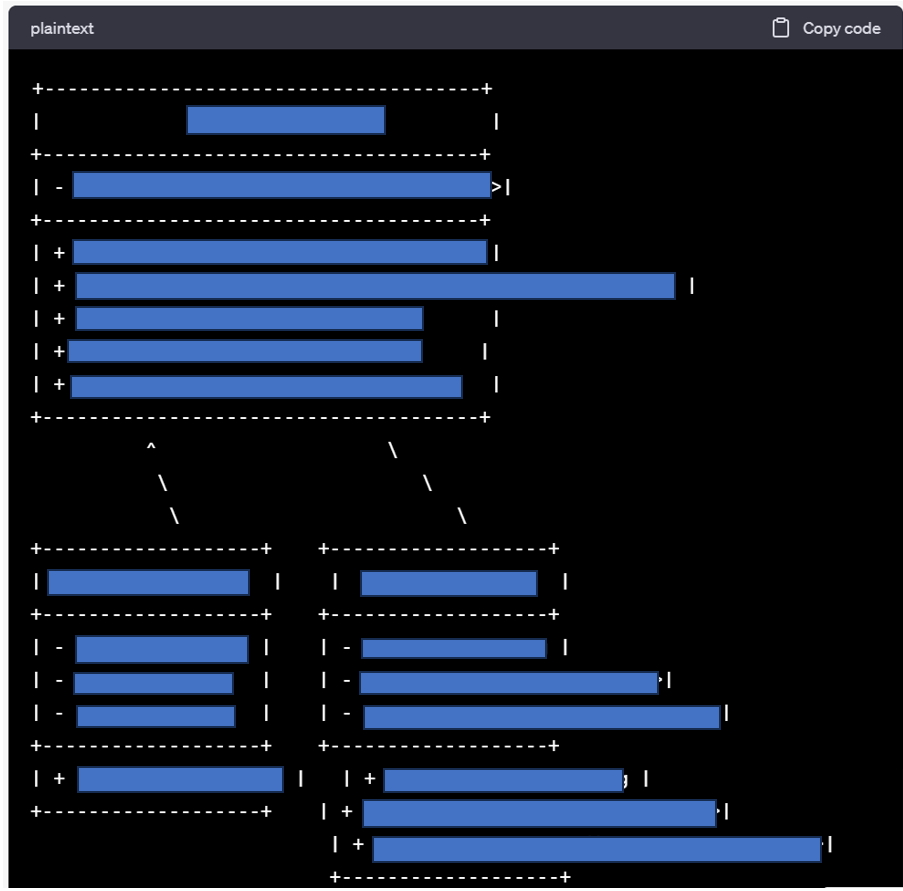
References:
Alkaissi, H., & McFarlane, S. I. (2023). Artificial hallucinations in ChatGPT: implications in scientific writing. Cureus, 15(2).
Smulders, S. (2023, March 29). 15 rules for crafting effective GPT Chat prompts. Expandi. https://expandi.io/blog/chat-gpt-rules/