There are many AI applications for image generation, but many of them are based on the Internet and the cloud, and are charged by subscription or based on the number of times used. Unlike these, Stable Diffusion WebUI, as an open source and free image generation tool, has attracted widespread attention with its powerful capabilities. However, it also has a relatively high threshold for use. Based on my experience in using Stable Diffusion WebUI, I will briefly talk about the potential commercial prospects of low-threshold, easy-to-use local image generation AI applications.
Advantages of Stable Diffusion WebUI
The advantage of Stable Diffusion WebUI is not only that it is completely open source and free, but also that its model is deployed locally (that is, similar to the end-side AI mentioned at the iPhone16 conference). Since it runs directly on local hardware, it does not need to upload any data to the cloud. This means that users can fully call on the computing resources of their own devices without connecting to the network.
Compared with cloud applications such as MidJourney, the protection of data privacy is a major advantage of local applications. Neither the user’s input nor the generated images are uploaded to the server, but are processed locally, which is suitable for users who are sensitive to data security and privacy.
At the same time, because it runs on local hardware, its performance can be very high. It can flexibly call on the computing power of a high-end GPU, which is especially suitable for users with high performance hardware. It is not affected by network bandwidth and gives full play to its powerful image generation capabilities. All this makes it an extremely excellent tool.
The Threshold of Using Stable Diffusion WebUI
Although Stable Diffusion WebUI is powerful, its threshold of use also makes many ordinary users discouraged. This is my personal experience in installation, model import and debugging parameters.
First of all, the installation and operation process is relatively complicated. You need to download tools and deal with many environmental dependencies, such as Python and other necessary libraries. These steps are relatively complicated for many new users. Without the help of various forums, blogs and GPT, I would definitely not be able to do it.
In addition, the selection and import of models is also a big challenge. Although there are a large number of free model resources on the Internet, it is not easy to find a model that suits your needs. It will also take a lot of time to choose the SORA model. In the end, you need to adjust many parameters including the number of steps, sampling method, and resolution by yourself to achieve the desired effect. Its complexity also makes it difficult for users to control.
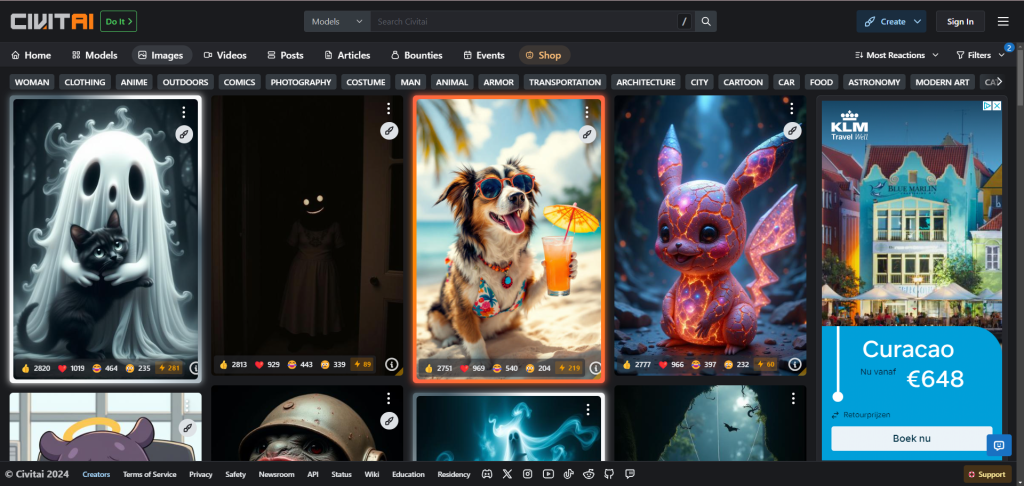
Launch Simple Applications to Attract More Users
If we can launch a simpler and easier-to-use app based on Stable Diffusion WebUI, which is aimed at the consumer market and the general public, we will be able to push it to a wider market.
The key is to lower the threshold for use. By integrating environmental dependencies, such as pre-installing all necessary libraries and operating environments, users can skip the configuration process. The application can also provide a one-click model download and purchase channel to help users quickly obtain high-quality generated models.
At the same time, user-friendly interface design is also essential. While following various interactive design principles, optimize UI and UX, and simplify complex parameter adjustments into several key options. Let ordinary users easily generate the pictures they want without losing flexibility. For example, users can control the quality and style of the generated pictures through simple sliders or preset modes, avoiding complex technical details.
Commercial Potential
From a commercial perspective, local image generation applications based on Stable Diffusion WebUI have broad prospects.
The use of Stable Diffusion WebUI not only avoids the occupation of cloud resources, but also makes pricing more flexible. Compared with the payment model of conventional online image generation AI such as MidJourney, Stable Diffusion’s local application can adopt a variety of pricing strategies, such as buying out software, paying for advanced models, or allowing users to use the generation service unlimited times within a certain period of time through a subscription system.
Overall, through the simplified operation experience and flexible and low pricing, we may be able to build an “unpopular” image generation AI application based on the Stable Diffusion WebUI, attracting a wide audience and looking forward to its large-scale application.